TerraMind
Large-scale generative multimodal deep learning model for EO. Released in open source.
The new foundation model from the FAST-EO project funded by the ESA Φ-lab combines insights from nine types of Earth observation data to provide an intuitive understanding of our planet.
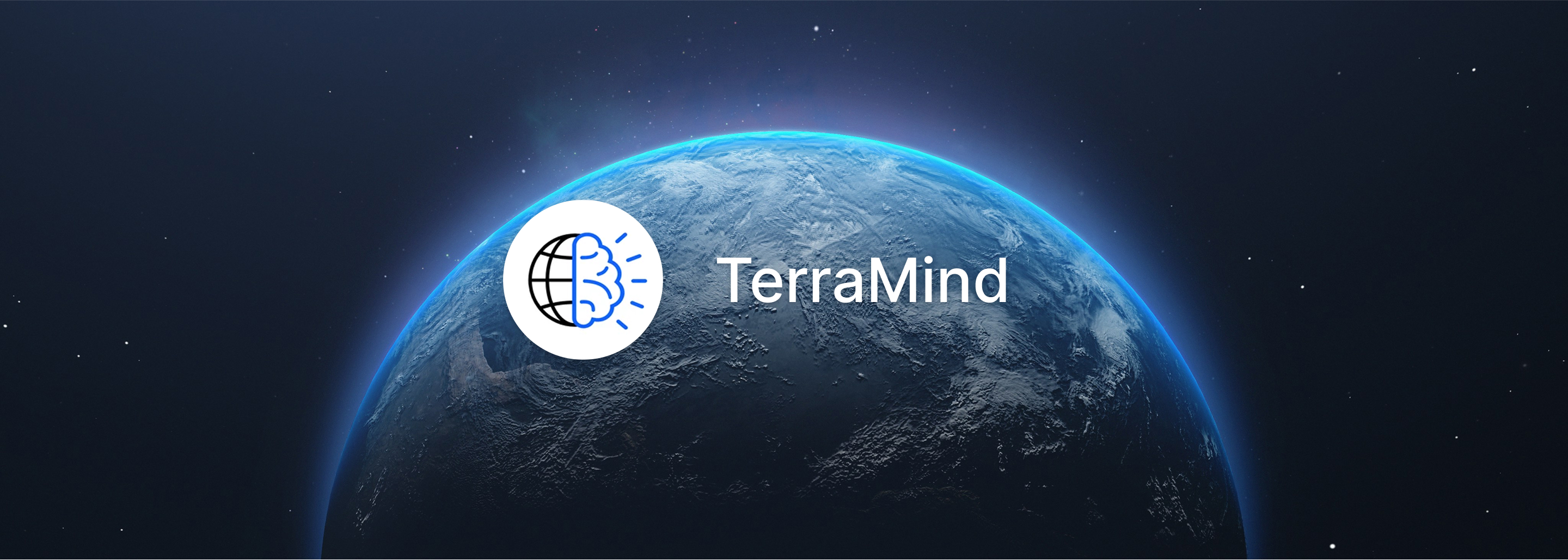
Key model features
TerraMind is groundbreaking even beyond the domain of Earth observation. It is the first “any-to-any” multi-modal generative AI model for Earth observation. This means it can self-generate additional training data from other modalities — a technique coined by FAST-EO researchers as “Thinking-in-Modalities” (TiM) tuning.
What information would an AI model need to truly understand our planet? The FAST-EO project set out to answer this question and to improve access to foundational models within the Earth-observation community. TerraMind is the new Earth-observation model that FAST-EO has open-sourced on Hugging Face. It was pre-trained on TerraMesh, the largest geospatial dataset available, which was built as part of the project.
A leader in geospatial model performance
TerraMind features a symmetric transformer-based encoder-decoder architecture engineered to handle pixel-based, token-based, and sequence-based inputs and to learn correlations across all three modalities. Although it was trained on 500 billion tokens, TerraMind remains compact and lightweight, requiring ten times less compute than using separate standard models for each modality. As a result, users can deploy it at scale at lower cost and with significantly reduced energy consumption at inference time.
“To me, what sets TerraMind apart is its ability to go beyond simply processing earth observations with computer vision algorithms. It instead has an intuitive understanding of geospatial data and our planet. At present, TerraMind is the best performing AI foundation model for Earth observation according to well-established community-benchmarks,” said Juan Bernabé-Moreno, director of IBM Research UK and Ireland, and IBM's Accelerated Discovery lead for climate and sustainability.
In an ESA evaluation, TerraMind was compared against 12 popular Earth observation foundation models on PANGAEA, a community-standard benchmark, to measure the model’s performance on real-world tasks, like land cover classification, change detection, environmental monitoring and multi-sensor and multi-temporal analysis. The benchmark showed TerraMind outperformed other models on these tasks by 8% or more.
“TerraMind combines insights from several modalities of training data to increase the accuracy of its outputs, the ability to intuitively bring in contextual information and generate unseen scenarios is a critical step in unlocking the value of ESA data. Compared to competitive models, it can uncover a deeper understanding of the Earth for researchers and businesses alike.” said Simonetta Cheli, director of ESA Earth Observation Programmes and Head of ESRIN.
In practice, to predict the risk of water scarcity, researchers need to consider many different factors like land use, climate, vegetation, agricultural activities, and location. Before TerraMind, all of this data was locked away in separate places. Bringing this information together enables users to predict the potential risk of water scarcity informed by a larger, more accurate picture of conditions on Earth.
Nine million data points, nine different modalities
During the dataset creation, researchers included data from all biomes, land use/land cover types, and regions, allowing the model to be equally applicable to any use case across the globe, with limited bias.
The dataset includes 9 million globally distributed, spatiotemporally aligned data samples across nine core data modalities – including observations made by sensors on satellites, the geomorphology of the Earth’s surface, surface characteristics that are important to life on Earth (vegetation and land use) and the basics of how to describe locations and features (latitude, longitude, and simple text descriptions).
Self-tuning to create artificial data
From a technical perspective, TerraMind is groundbreaking even beyond the domain of Earth observation. It is the first “any-to-any” multi-modal generative AI model for Earth observation. This means it can self-generate additional training data from other modalities — a technique IBM researchers coined “Thinking-in-Modalities” (TiM) tuning. TiM is a novel approach for computer vision models similar to chain-of-thought in language models. Empirical evidence demonstrates that TiM tuning can enhance the model performance beyond normal fine-tuning.
“TiM tuning boosts data efficiency by self-generating the additional training data relevant to the problem being addressed — for example, by telling the model to “think” about land cover when mapping water bodies. This breakthrough can unlock unprecedented accuracy when specializing TerraMind for particular use cases,” said Johannes Jakubik, an IBM Research scientist based in Zurich.
Try TerraMind for yourself
TerraMind is an open-source foundation model for geospatial data developed in the FAST-EO project. Get it now on Hugging Face and start testing it today!

Publications
J. Jakubik, F. Yang, B. Blumenstiel, E. Scheurer, R. Sedona, S. Maurogiovanni, J. Bosmans, N. Dionelis, V. Marsocci, N. Kopp, R.Ramachandran, P. Fraccaro, T. Brunschwiler, G.Cavallaro, J. Bernabe-Moreno, N. Longépé, "TerraMind: Large-Scale Generative Multimodality for Earth Observation", in arXiv:2504.11171, 2025, https://arxiv.org/abs/2504.11171
B. Blumenstiel, P. Fraccaro, V. Marsocci, J. Jakubik, S. Maurogiovanni, M. Czerkawski, R. Sedona, G.Cavallaro, T. Brunschwiler, J. Bernabe-Moreno, N. Longépé, "TerraMesh: A Planetary Mosaic of Multimodal Earth Observation Data" in arXiv:2504.11172, 2025, https://arxiv.org/abs/2504.11172
"IBM, ESA Launch TerraMind, New AI Model for Earth and Climate Monitoring", ESG News, April 22, 2025
https://esgnews.com/ibm-esa-launch-terramind-new-ai-model-for-earth-and-climate-monitoring/
"IBM and ESA open-source TerraMind, the best performing generative AI model for Earth observation", IBM Blog, April 22, 2025, https://research.ibm.com/blog/terramind-esa-earth-observation-model
"TerraMind: A Breakthrough Multimodal Model for Global-Scale Earth Observation", Jülich Supercomputing Centre News, April 22, 2025, https://www.fz-juelich.de/en/ias/jsc/news/news-items/news-flashes/2025/terramind-a-breakthrough-multimodal-model-for-global-scale-earth-observation