Use case applications for FAST-EO
Fostering Advancements in Foundation Models via Unsupervised and Self-supervised Learning for Downstream Tasks in Earth Observation

Weather & Climate Disaster Analysis
Fine-tune Geospatial Foundation Models for global natural disaster segmentation and impact assessment using multi-satellite data to improve disaster management and risk estimation.
Obtain pixel-level segmentation of natural disasters (floods, wildfire scars) from multi-satellite data (Sentinel-1 and Sentinel-2).
Provide textual descriptions of disaster impacts, enhancing disaster response and risk management.

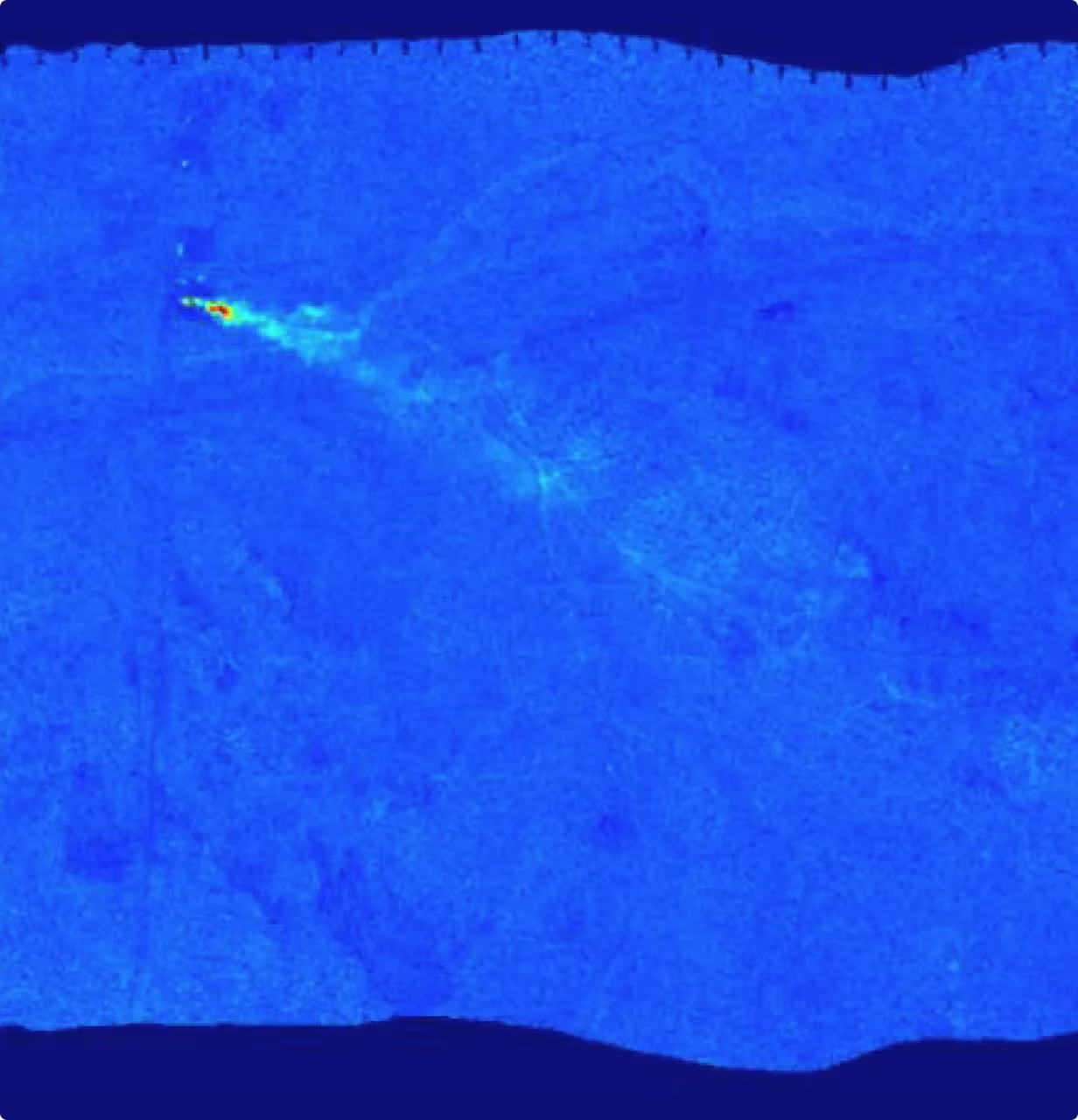
Detection of Methane Leaks
Fine-tuning of geospatial foundation models to detect methane emissions using satellite data. This use case involves analyzing spectral data and employing high-resolution images to identify methane leaks accurately.
Develop an AI system to detect methane emissions using satellite data, analyzing spectral data and employing high-resolution images for accurate identification of methane leaks.
Enable practical analysis of methane leaks to allow for more accurate predictions of their effects and reduce their impact on climate change.
Observation of Changes in Forest Above-Ground Biomass
Fine-tuning of geospatial foundation models to retrieve and analyze forest metrics such as basal area, tree cover density, and tree heights, and detect changes over time, including their causes, to support forest management and ecological monitoring.
Retrieve forest basal area, tree cover density, and tree heights, and detect changes compared to a baseline scenario, including identifying the causes of these changes.
Deliver accurate, consistent, and frequently updated forest metrics across diverse landscapes, improving forest management and ecological monitoring.
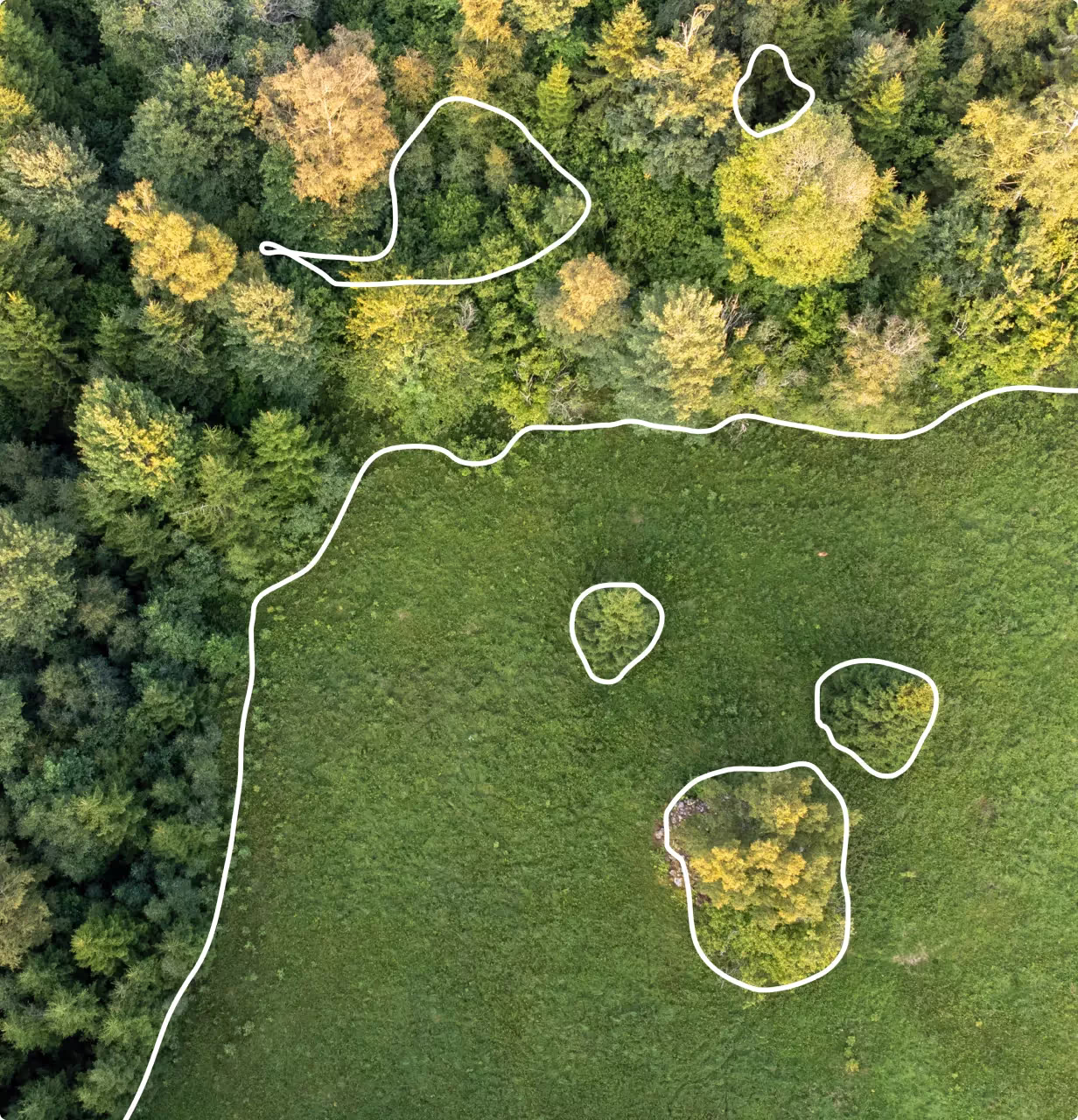
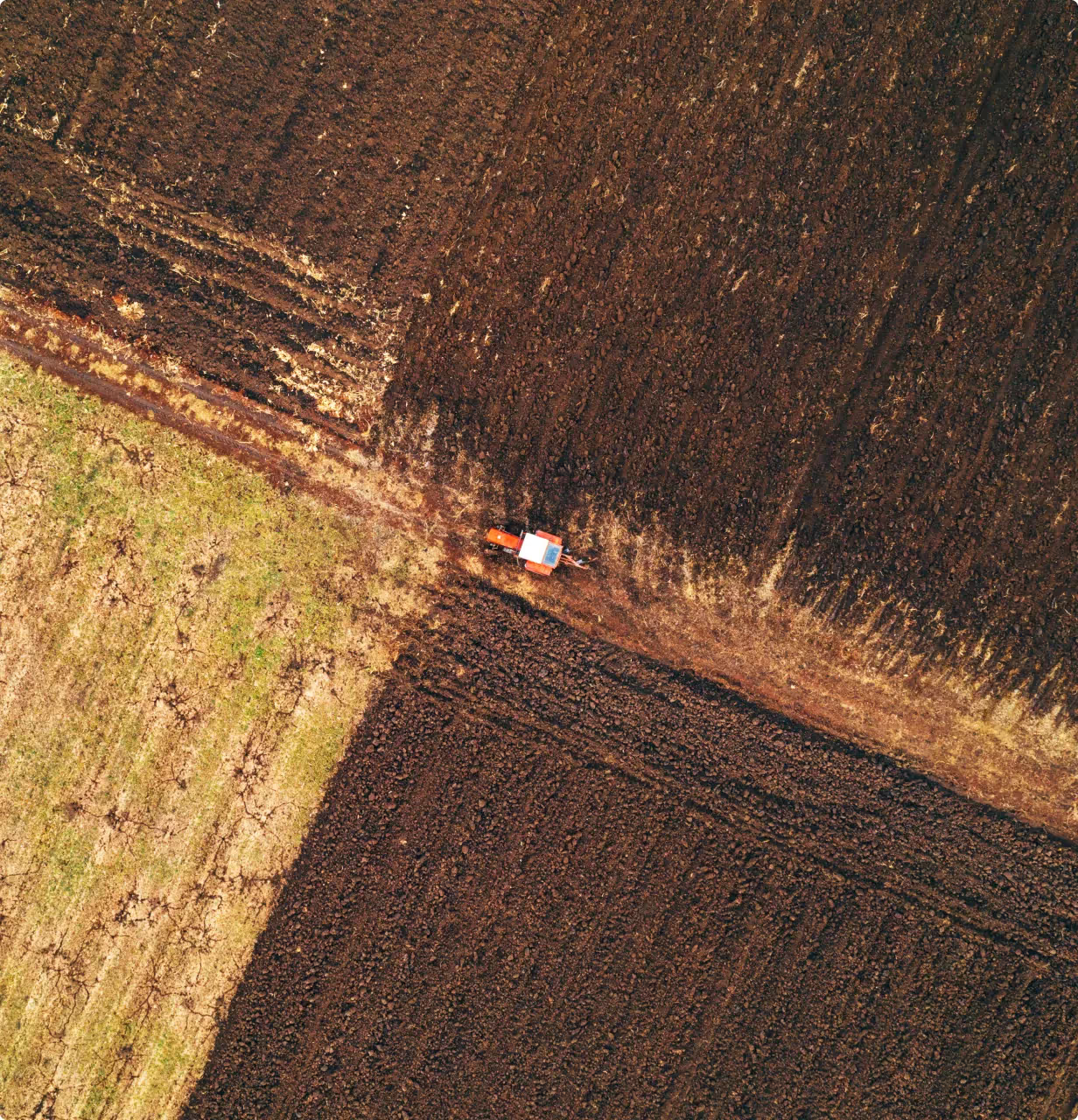
Estimation of Soil Properties
Fine-tuning of geospatial foundation models to determine soil properties from hyperspectral imagery. This use case promises to transform soil analysis, making it possible to assess soil conditions over large areas more efficiently and accurately.
Use AI to determine soil properties from hyperspectral imagery, transforming soil analysis for efficient and accurate large-area assessments.
Enable local governments and regions to organize public policies related to agriculture and forestry, and assess the necessity and justification for support in these areas.
Detection of Semantic Land Cover Changes
Fine-tuning of geospatial foundation models to develop reliable and consistent land cover maps to monitor multi-year patterns across large-scale areas, aiding in the detection of dynamic changes due to human activities and natural disasters, while leveraging advanced remote sensing data for frequent updates.
Create reliable and consistent land cover maps using remote sensing data from Sentinel-1 and 2 to monitor multi-year patterns across Europe.
Continuously update land cover maps to detect and analyze changes due to extreme weather events, natural disasters, urban development, and other human activities, providing valuable insights for researchers and policymakers.
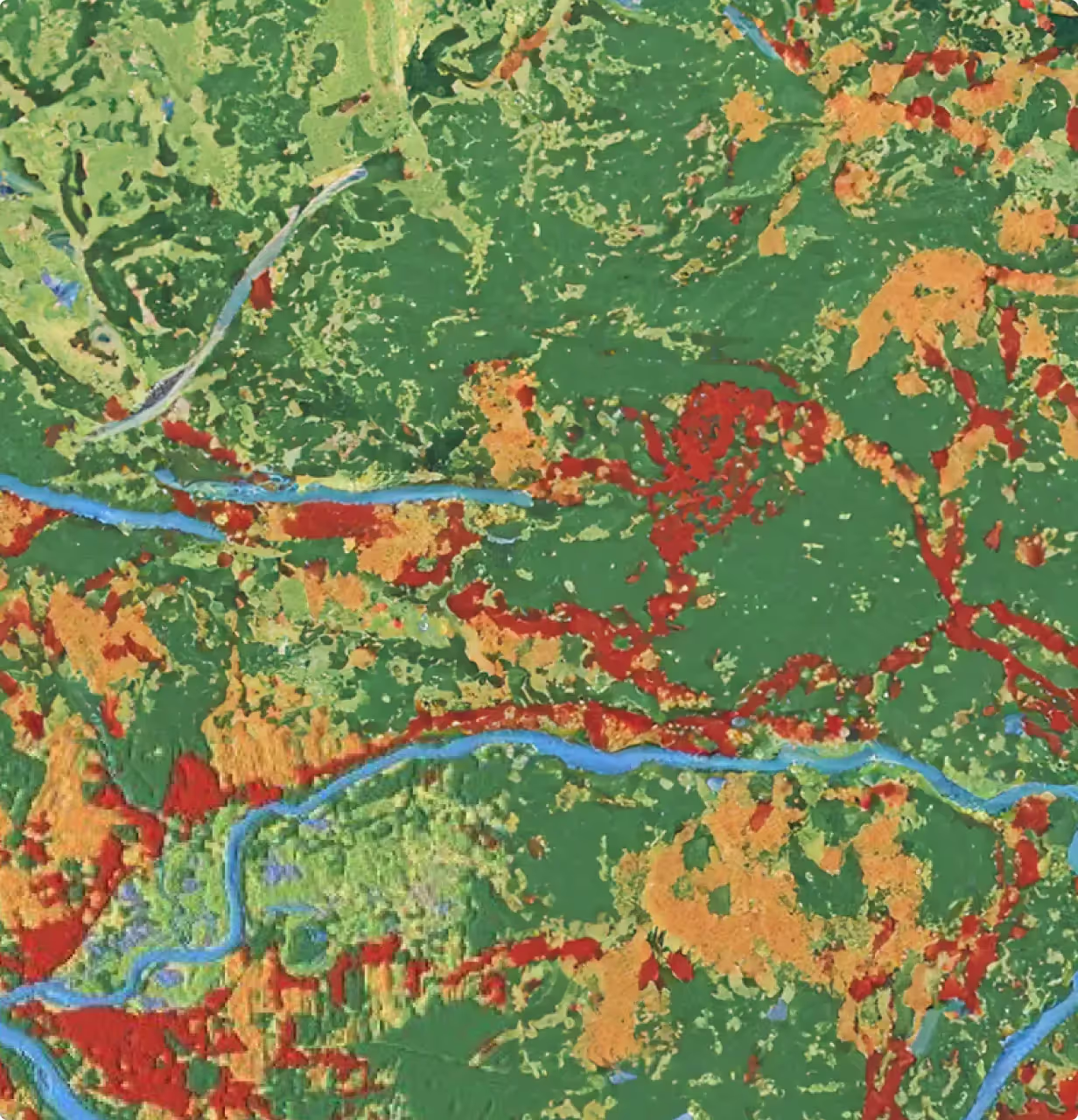

Monitoring Expansion of Mining Fields into Farmlands
Fine-tuning of geospatial foundation models to examine the effects of mining expansion on Ghanaian forests, with a specific focus on cocoa croplands. This approach leverages Sentinel-1 and Sentinel-2 data to track spatial dynamics and assess environmental changes, supporting regulatory efforts and policy evaluation.
Developing a benchmark dataset that integrate publicly available data with information provided by local farmers (Amansie West district).
Examination of the impact of EO data type on feature embeddings, aimed at gaining deeper insights into how foundation models learn and represent the intrinsic characteristics of mining fields and agricultural lands in different observation scenarios.