Addressing Societal Challenges with Earth Observation and Foundation Models

Democratizing Foundation Models for Earth Observation Applications
A. Zappacosta, R. S. Kuzu, C. O. Dumitru, D. E. Molina, T. Brunschwiler, R. Kienzler, J. Jakubik, B. Blumenstiel, G. Cavallaro, S. Kesselheim, R. Sedona, A. Wijata, L. Tulczyiew, D. Marek, J. Nalepa
In Earth Observation (EO), integrating Foundation Models (FMs) poses a challenge due to disparities with conventional computer vision tasks, particularly in processing multispectral/hyperspectral data. Additionally, the high computational resources needed for FM training limit their widespread use. Leveraging state-of-the-art FMs, we extend their utility to suit diverse sensor types, temporal characteristics, and domains, enhancing their applicability in various EO tasks. Our approach offers a flexible solution, accommodating different multi-modal FMs, and extends beyond specific models for broader EO applications. To demonstrate the effectiveness of this framework, we explore diverse EO use cases (UCs), spanning different thematic areas and geographic locations, and showcase the framework's potential for generalization and adaptability across a range of EO challenges, as part of the FAST-EO project.
Addressing Societal Challenges with Earth Observation and Foundation Models
A. Zappacosta, S. Ofori-Ampofo, R. S. Kuzu, D. E. Molina
The rapid growth of data in today's era has increased the complexity of data-driven issues in science and engineering. This has led to a paradigm shift in Artificial Intelligence (AI), with a focus on unsupervised/self-supervised learning for vast data volumes, and multimodal learning for integrating diverse data sources, enhancing decision-making and insights across various applications. Embracing this AI shift for societal challenges, DLR, IBM, FZJ, and KP Labs have proposed a Geo-foundation Model framework, FAST-EO, for multiple applications. This manuscript introduces two of these applications, to be provided by DLR, targeting specific challenges in agriculture and forestry.
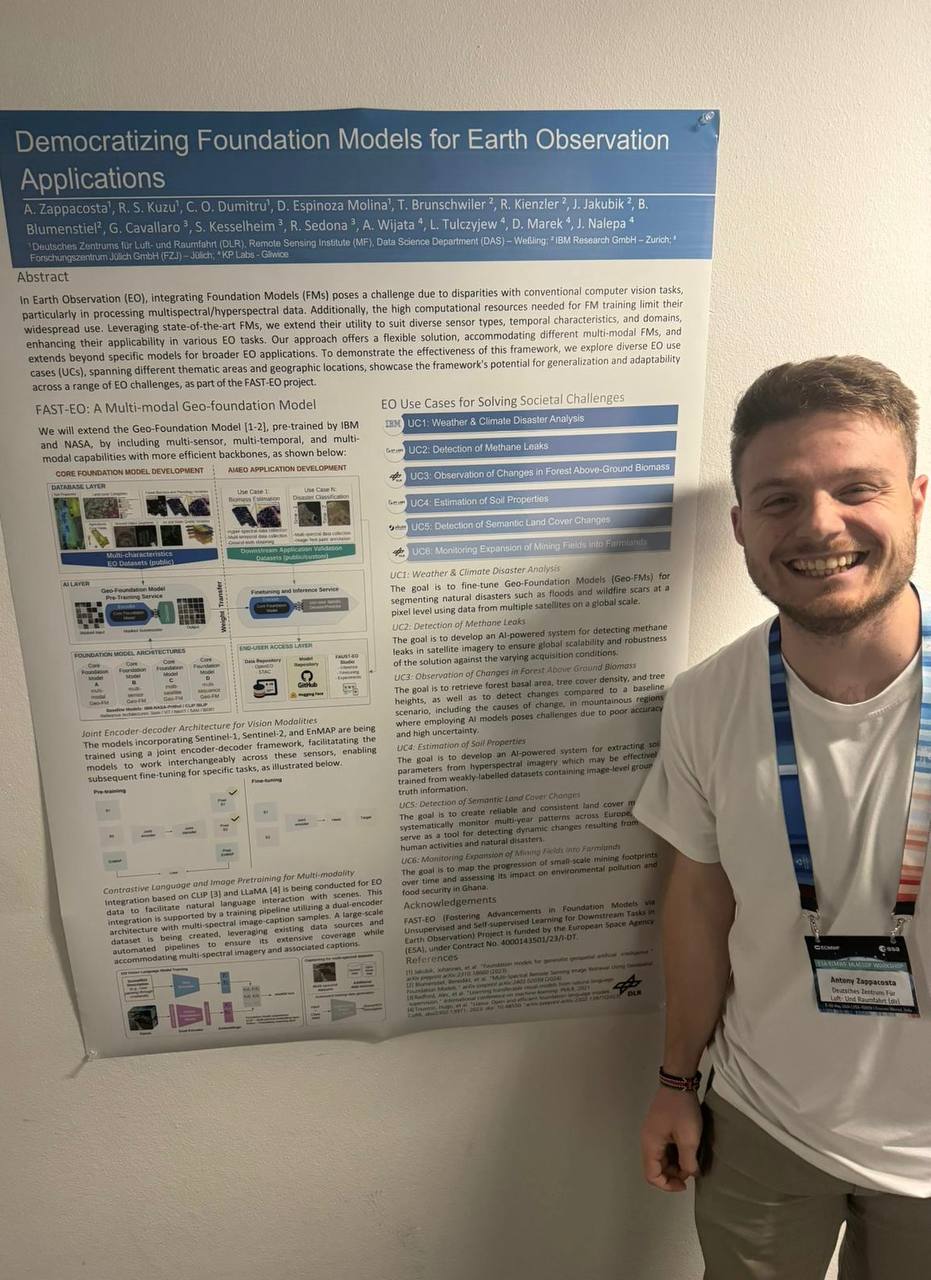